Principles of Modeling Uncertainties in Spatial Data and Spatial Analyses

In the realm of spatial data and spatial analyses, uncertainty is an ever-present challenge. Spatial data, often collected from diverse sources and representing complex phenomena, is inherently uncertain. This uncertainty can propagate through spatial analyses, potentially leading to unreliable or misleading results.
To address this challenge, researchers and practitioners have developed a range of techniques for modeling uncertainties in spatial data and spatial analyses. These techniques empower us to quantify and account for uncertainty, enabling us to make more informed decisions in the face of data variability and uncertainty.
4.3 out of 5
Language | : | English |
File size | : | 17269 KB |
Screen Reader | : | Supported |
Print length | : | 432 pages |
Types of Uncertainties in Spatial Data
Uncertainties in spatial data can arise from various sources, including:
- Measurement error: Errors introduced during data collection, such as inaccuracies in measurements or instrument limitations.
- Sampling error: Uncertainties due to the selection of a limited number of samples to represent a larger population.
- Model error: Uncertainties resulting from the use of simplified models to represent complex real-world phenomena.
- Data uncertainty: Uncertainties inherent in the data itself, such as missing or incomplete data, or ambiguities in data interpretation.
Principles of Uncertainty Modeling
Uncertainty modeling aims to quantify and represent the uncertainties associated with spatial data and spatial analyses. Key principles include:
- Uncertainty propagation: Tracking how uncertainties in input data propagate through spatial analyses, allowing for the assessment of overall uncertainty in the results.
- Uncertainty visualization: Representing uncertainties in a visual and intuitive manner, facilitating the communication and interpretation of results.
- Uncertainty analysis: Evaluating the impact of uncertainties on decision-making, providing insights into the robustness and sensitivity of spatial analyses to uncertainty.
Techniques for Uncertainty Modeling
Various techniques are employed for uncertainty modeling in spatial data and spatial analyses, including:
- Error propagation: Propagating uncertainties through spatial analyses using error propagation equations, allowing for the quantification of uncertainty in the output.
- Monte Carlo simulation: Generating multiple realizations of spatial data and spatial analyses, providing a probabilistic representation of uncertainty.
- Fuzzy logic: Representing uncertainty using fuzzy sets, allowing for the gradation of uncertainty levels and the modeling of imprecise or subjective information.
- Bayesian analysis: Incorporating prior knowledge and data to update beliefs about uncertainty, enabling probabilistic inference and decision-making under uncertainty.
Applications of Uncertainty Modeling
Uncertainty modeling has a wide range of applications in spatial data and spatial analyses, including:
- Environmental modeling: Quantifying uncertainties in climate models, pollution dispersion models, and natural hazard risk assessments.
- Health risk assessment: Assessing uncertainties in exposure models and health impact assessments, informing decision-making related to public health.
- Land use planning: Identifying uncertainties in land use change models, supporting informed land use decisions and sustainable development.
- Transportation planning: Evaluating uncertainties in traffic models and transportation network analysis, optimizing transportation systems and reducing congestion.
Modeling uncertainties in spatial data and spatial analyses is a critical aspect of ensuring the reliability and usefulness of spatial information. By understanding the principles and techniques of uncertainty modeling, we can effectively quantify and account for uncertainties, enabling us to make more informed decisions and mitigate the risks associated with uncertain information. As the field of spatial data and spatial analyses continues to evolve, advancements in uncertainty modeling will play a crucial role in enhancing our ability to address complex spatial problems and make data-driven decisions in the face of uncertainty.
4.3 out of 5
Language | : | English |
File size | : | 17269 KB |
Screen Reader | : | Supported |
Print length | : | 432 pages |
Do you want to contribute by writing guest posts on this blog?
Please contact us and send us a resume of previous articles that you have written.
Book
Novel
Page
Chapter
Text
Story
Genre
Reader
Library
Paperback
E-book
Magazine
Newspaper
Paragraph
Sentence
Bookmark
Shelf
Glossary
Bibliography
Foreword
Preface
Synopsis
Annotation
Footnote
Manuscript
Scroll
Codex
Tome
Bestseller
Classics
Library card
Narrative
Biography
Autobiography
Memoir
Reference
Encyclopedia
Joel Stevens
Jessica Caplain
John Bryan Starr
Jim Gorant
Pamela K Keel
Robin Sturtz
Yingchi Chu
Leon King
John Keegan
Nathaniel Bluedorn
Thomas Forrest Kelly
John M Doris
John Jung
Richard Jensen
Xing Lu
Jennifer Moreau
Marisa Moris
Jessica Matthews
Peter B Orlik
Joe Laredo
Light bulbAdvertise smarter! Our strategic ad space ensures maximum exposure. Reserve your spot today!
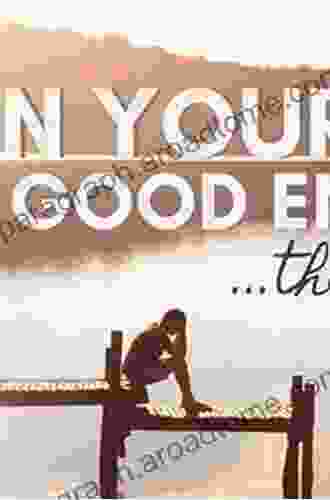

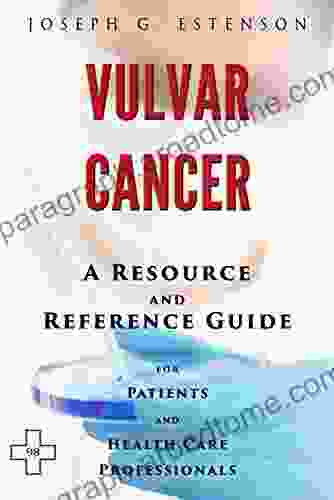

- Christian BarnesFollow ·5.7k
- Heath PowellFollow ·8.1k
- Robert FrostFollow ·19.6k
- Dawson ReedFollow ·3.7k
- Colby CoxFollow ·13.8k
- Holden BellFollow ·7.6k
- Braden WardFollow ·3.1k
- Wade CoxFollow ·9k
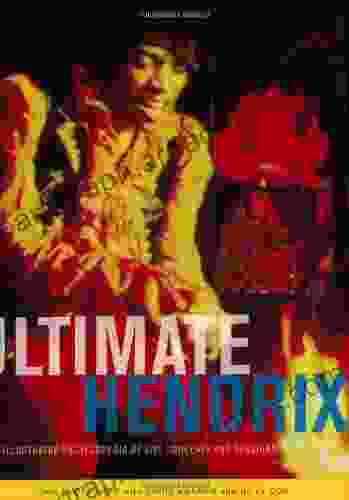

An Illustrated Encyclopedia Of Live Concerts And...
Immerse yourself in the...
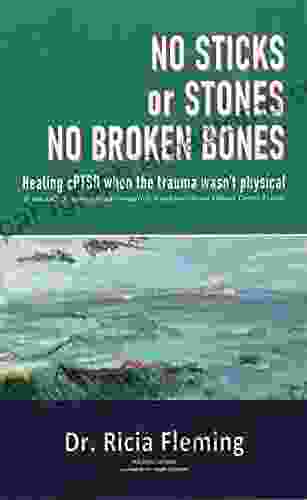

Non Physically Assaultive Attachment Based Chronic Covert...
What is Covert...
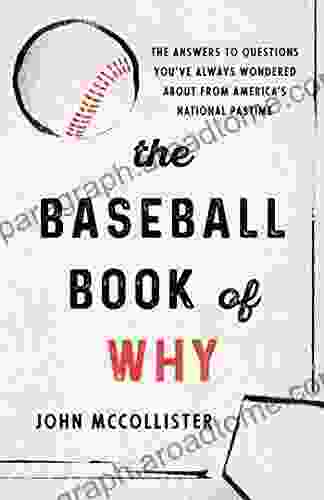

The Baseball of Why: Unraveling the Intricacies of...
Step up to the plate and...
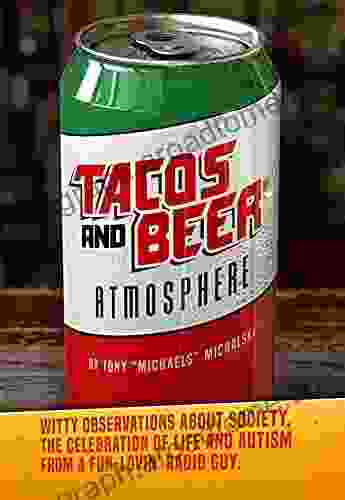

Tacos and Beer: An Atmosphere of Flavorful Delights
In the realm of culinary adventures,...
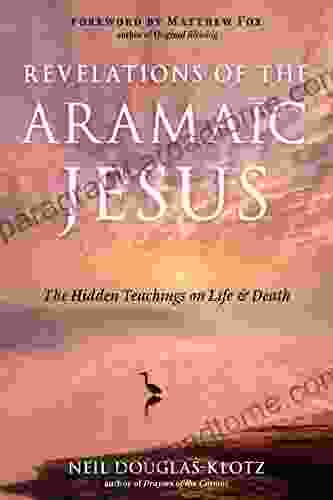

Unlock the Secrets of the Aramaic Jesus: Revelations of a...
Journey Back to the Roots of...
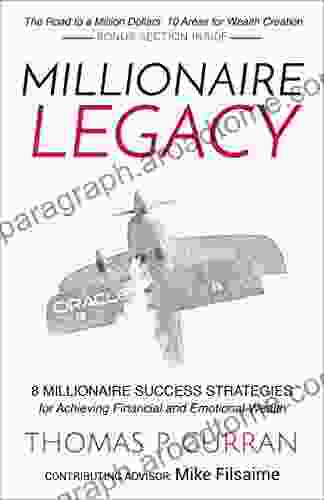

Millionaire Success Strategies: Your Blueprint for...
Unlock the...
4.3 out of 5
Language | : | English |
File size | : | 17269 KB |
Screen Reader | : | Supported |
Print length | : | 432 pages |